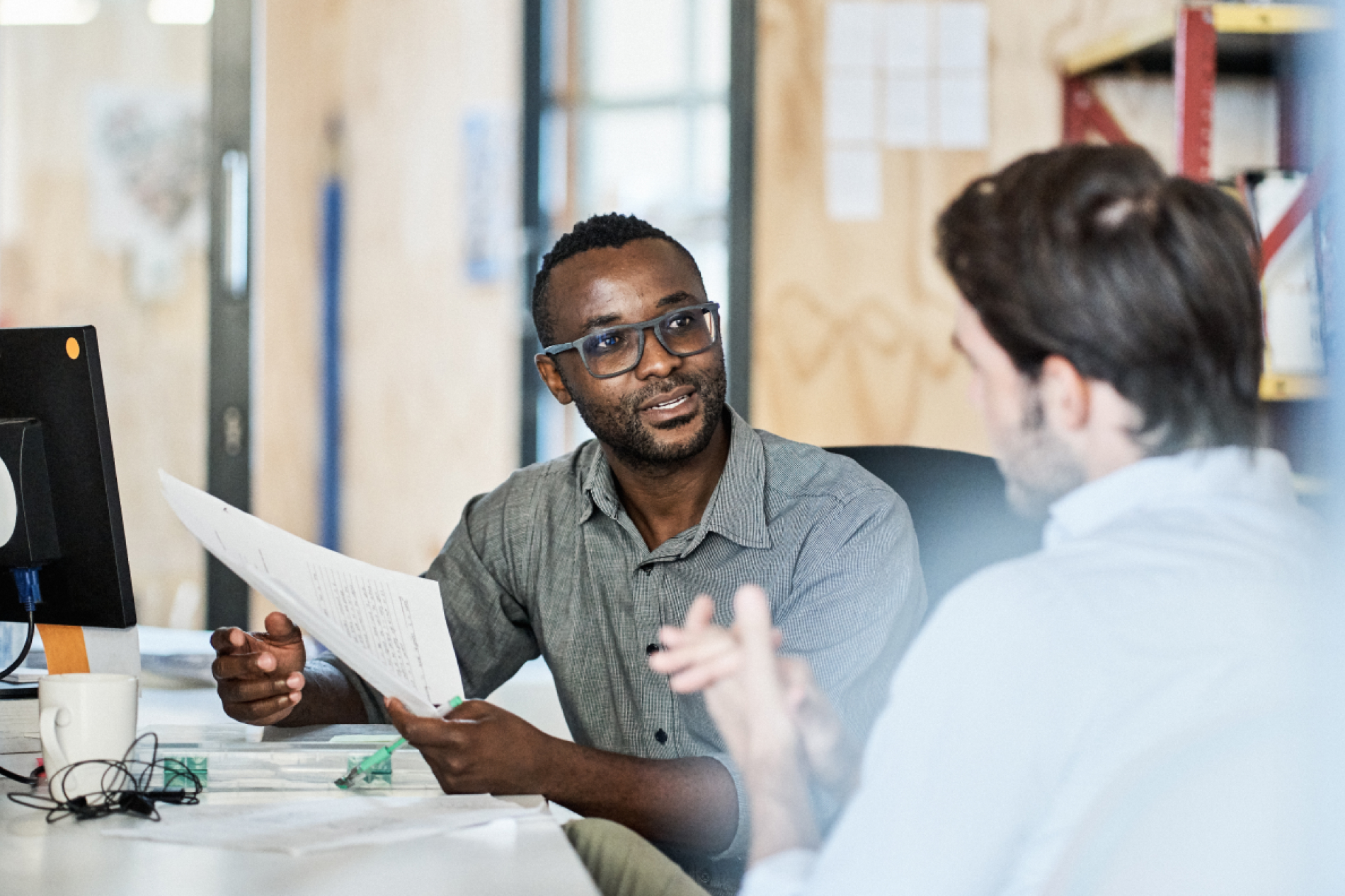
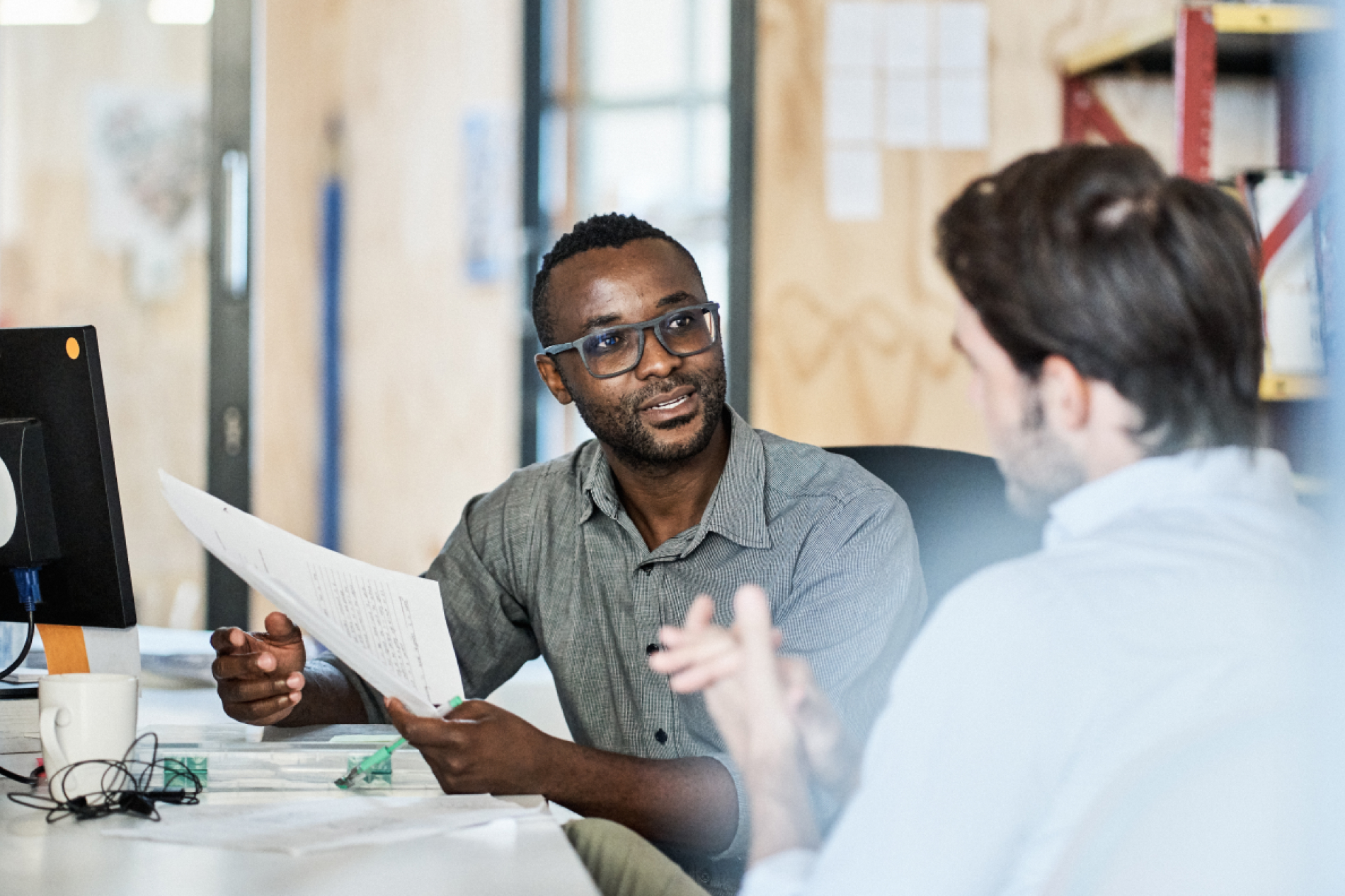
By Michael Kanellos, Head of Influencer Relations, Marvell
Bigger is better, right? Look at AI: the story swirls with superlatives.
ChatGPT landed one million users within five days,1 far surpassing the pace of any previous technology. The compute requirements of training notable AI models increases 4.5x per year while training data sets mushroom by 3x per year,2 etc.
Bigger, however, comes at a price. Data center power consumption threatens to nearly triple by 2028 primarily because of AI3. Water withdrawals, meanwhile, are escalating as well: by 2027, AI data centers could need up to 6.6 billion cubic meters, or about half of what the UK uses.4 The economic and environmental toll over the long run may not be sustainable.
Conceptually it is easier to understand how larger models translate into a "better and more capable" model. The more layers or parameters the models have, contribute to the quality and accuracy of the model. Yet, can we sustain that extracted value at the same cadence by continuing the size increase? Or will the curve start to plateau at some point?
By Nick Kucharewski, Senior Vice President and General Manager, Cloud Platform Business Unit, Marvell
The rapid expansion in the size and capacity of AI workloads is significantly impacting both computing and network technologies in the modern data center. Data centers are continuously evolving to accommodate higher performance GPUs and AI accelerators (XPUs), increased memory capacities, and a push towards lower latency architectures for arranging these elements. The desire for larger clusters with shorter compute times has driven heightened focus on networking interconnects, with designers embracing state-of-the-art technologies to ensure efficient data movement and communication between the components comprising the AI cloud.
A large-scale AI cloud data center can contain hundreds of thousands, or millions, of individual links between the devices performing compute, switching, and storage functions. Inside the cloud, there is a tightly ordered fabric of high-speed interconnects: webs of copper wire and glass fiber each carrying digital signals at roughly 100 billion bits per second. Upon close inspection there is a pattern and a logical ordering to each link used for every connection in the cloud, which can be analyzed by considering the physical attributes of different types of links.
For inspiration, we can look back 80 years to the origins of modern computing, when John von Neumann posed the concept of memory hierarchy for computer architectures1. In 1945 Von Neumann proposed a smaller faster storage memory placed close to the compute circuitry, and a larger slower storage medium placed further away, to enable a system delivering both performance and scale. This concept of memory hierarchy is now pervasive, with the terms “Cache”, “DRAM”, and “Flash” part of our everyday language. In today’s AI cloud data centers, we can analyze the hierarchy of interconnects in much the same way. It is a layered structure of links, strategically utilized according to their innate physical attributes of speed, power consumption, reach, and cost.
The hierarchy of interconnects
This hierarchy of interconnects provides a framework for understanding emerging interconnect technologies and to assess their potential impact in the next generation of AI data centers. Through a discussion of the basic attributes of emerging interconnect technologies in the context of the goals and aims of the AI cloud design, we can estimate how these technologies may be deployed in the coming years. By identifying the desired attributes for each use case, and the key design constraints, we can also predict when new technologies will pass the "tipping point" enabling widespread adoption in future cloud deployments.
Copyright © 2025 Marvell, All rights reserved.